From chemical Graphs To Structures
28. Juli 2021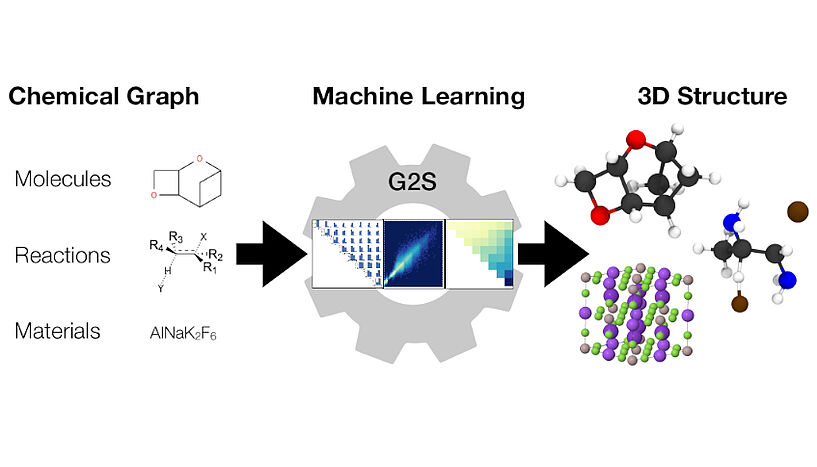
The machine learning model Graph2Structure uses graphs of chemical compounds (left) to predict their 3D coordinates (right). (© Dominik Lemm, University of Vienna)
Three-dimensional (3D) configurations of atoms dictate all materials properties. Quantitative predictions of accurate equilibrium structures, 3D coordinates of all atoms, from a chemical graph, a representation of the structural formula, is a challenging and computationally expensive task which is at the beginning of practically every computational chemistry workflow. Researchers at the University of Vienna have now developed a new machine learning based model to shortcut expensive calculations to directly predict structures from graphs. The new method for "Machine learning based energy-free structure predictions of molecules, transition states, and solids" is presented in the latest issue of Nature Communications.
Albeit commonly depicted as rigid, chemical compounds are flexible three dimensional objects made up of atoms which continuously move and oscillate. Cyrus Levinthal noted already in 1969 that the large amount of degrees of freedom of chemical compounds formally leads to a catastrophically large number of possible conformations well up to 10300 (Levinthal’s Paradoxon). Within experimental observations, however, 3D configurations of atoms correspond to well defined free energy minima and thereby dictate all materials properties. The paradigm that structure determines function is key for determining drug interactions, optimizing catalysts or reactions, and materials discovery. As a consequence, in most computational high throughput screening campaigns (a method for rapid scientific experimentation), only the most stable configurations are sought after. Depending on the level of sophistication within the approximations made when estimating materials’ stabilities, computational cost can vary from minutes to hours or even days for the computation of a single structure. Given the vastness of chemical compound space, the space populated by all conceivable compounds (estimated to exceed 1060) this cost-quality tradeoff represents a major bottleneck in the field.
Researchers at the University of Vienna led by Anatole von Lilienfeld tackled this problem from a different perspective, developing a new method that leverages data and is universally applicable to any sort of chemistries. Their new method, Graph2Structure, uses high quality quantum chemical data in order to train machine learning models capable of predicting new 3D structures for molecular graphs of unseen compounds. This direct mapping of a molecular graph to a specific 3D configuration enables the model to effectively bypass any form of energy minimization, leading to a speedup of over a million when compared to the conventional methods. "The possibility of generating high quality structures does not only accelerate high throughput molecular design, but also accelerates the everyday workflow" – says lead author of the study in Nature Communications Dominik Lemm. "Reliably generating 3D structures for even exotic chemistries, such as open-shell systems or transition states, is one of the most difficult tasks in atomistic simulation". Further findings suggest that the generated structures can directly be used as an input to subsequent evaluation of machine learning based property prediction models, thereby linking a molecular graph to a structure dependent property in a rigorous and more effective way.
Publication in Nature Communications:
Machine learning based energy-free structure predictions of molecules, transition states, and solids
Dominik Lemm, Guido Falk von Rudorff, O. Anatole von Lilienfeld. DOI: 10.1038/s41467-021-24525-7
Wissenschaftlicher Kontakt
Univ.-Prof. Dr. Otto Anatole von Lilienfeld-Toal
Computergestützte Materialphysik Fakultät für Physik1090 - Wien, Kolingasse 14-16
+43-1-4277-51413
+43-664-60277-51413
anatole.vonlilienfeld@univie.ac.at
Rückfragehinweis
Pia Gärtner, MA
Pressebüro der Universität WienUniversität Wien
1010 - Wien, Universitätsring 1
+43-1-4277-17541
pia.gaertner@univie.ac.at
Downloads:
Unbenannt_13.jpg
Dateigröße: 101,6 KB